S&P 500 Stock Market Analysis
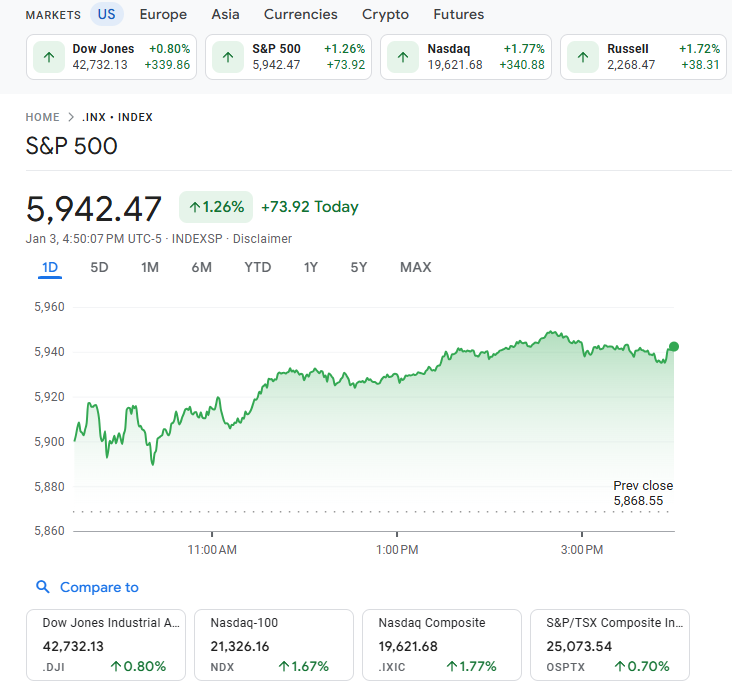
#data #dataanalysis #python #ETL
By Sergio Castro
About our dataset
Through this analysis we will be exploring a dataset that containts the 5-Year stock record of 500 companies.
The Standard and Poor’s 500, or simply the S&P 500 is a stock market index tracking the stock performance of 500 of the largest companies listed on stock exchanges in the United States. It is one of the most commonly followed equity indices and includes approximately 80% of the total market capitalization of U.S. public companies, with an aggregate market cap of more than $43 trillion as of January 2024.
This analysis has two main objectives: to explore the available data and create useful visualizations, as well as to identify any correlations between the stock prices of different companies.
We first import some packages that will help us throgh our analysis.
import pandas as pd
import numpy as np
import matplotlib.pyplot as plt
import seaborn as sns
import glob
We then connect our notebook to the local data we will be using to perform our stock analysis.
glob.glob(r'C:\Users\Sergio C\Desktop\Analisis\Stock analysis\2-Time Series Data Analysis\individual_stocks_5yr/*csv')
# Here we are adding a *csv so we can pull all our cvs files on the local path.
len(glob.glob(r'C:\Users\Sergio C\Desktop\Analisis\Stock analysis\2-Time Series Data Analysis\individual_stocks_5yr/*csv'))
509 # Here we are just checking how many files we have.
After connecting our notebook to the data in question, we create a list that will only include those specific companies records we are interested in. We can now can iniate to explore our data and store specific information we need into perfom calculations.
company_list = [
r'C:\\Users\\Sergio C\\Desktop\\Analisis\\Stock analysis\\2-Time Series Data Analysis\\individual_stocks_5yr\\AAPL_data.csv' ,
r'C:\\Users\\Sergio C\\Desktop\\Analisis\\Stock analysis\\2-Time Series Data Analysis\\individual_stocks_5yr\\AMZN_data.csv' ,
r'C:\\Users\\Sergio C\\Desktop\\Analisis\\Stock analysis\\2-Time Series Data Analysis\\individual_stocks_5yr\\GOOG_data.csv' ,
r'C:\\Users\\Sergio C\\Desktop\\Analisis\\Stock analysis\\2-Time Series Data Analysis\\individual_stocks_5yr\\MSFT_data.csv'
]
import warnings
from warnings import filterwarnings
filterwarnings('ignore') # This is just to ignore some warnings that may get our documents too long or harder to follow.
all_data = pd.DataFrame() #here we are creating a dataframe leveraging our pandas package and our company list previusly created (company_list).
for file in company_list:
current_df = pd.read_csv(file)
all_data = pd.concat([current_df , all_data] , ignore_index=True)
all_data.shape
(4752, 7)
all_data.head(6) # Some exploration to understand what kind of data we have available now.
date open high low close volume Name
0 2013-02-08 27.35 27.71 27.310 27.55 33318306 MSFT
1 2013-02-11 27.65 27.92 27.500 27.86 32247549 MSFT
2 2013-02-12 27.88 28.00 27.750 27.88 35990829 MSFT
3 2013-02-13 27.93 28.11 27.880 28.03 41715530 MSFT
4 2013-02-14 27.92 28.06 27.870 28.04 32663174 MSFT
5 2013-02-15 28.04 28.16 27.875 28.01 49650538 MSFT
all_data['Name'].unique() # We retrieve the unique values from the 'name' column to ensure it contains only the desired entries.
array(['MSFT', 'GOOG', 'AMZN', 'AAPL'], dtype=object)
Data Cleaning/Transformation
We ensure that our data is free of empty spaces and duplicate rows. Additionally, we double-check the data types to confirm accuracy and make necessary transformations when required.
all_data.isnull().sum() # checking empty rows
date 0
open 0
high 0
low 0
close 0
volume 0
Name 0
dtype: int64
all_data.dtypes # checking data types
date object
open float64
high float64
low float64
close float64
volume int64
Name object
dtype: object
all_data['date'] = pd.to_datetime(all_data['date']) # Transforming our Date column from "object" to datetime64[ns]
all_data['date']
0 2013-02-08
1 2013-02-11
2 2013-02-12
3 2013-02-13
4 2013-02-14
...
4747 2018-02-01
4748 2018-02-02
4749 2018-02-05
4750 2018-02-06
4751 2018-02-07
Name: date, Length: 4752, dtype: datetime64[ns] #Here we can see the dtype changed
We then create a list (‘tech_list’) of the stocks we are interested in analyzing, each identified by its specific ticker symbol, such as MSFT for Microsoft, GOOG for Google, AMZN for Amazon, and AAPL for Apple.
tech_list = all_data['Name'].unique()
tech_list
array(['MSFT', 'GOOG', 'AMZN', 'AAPL'], dtype=object)
With our data now organized and cleaned, we can leverage visualization libraries to create comparative visualizations of stock process over the years.
plt.figure(figsize=(20,12)) # Here we are comparing different closing prices by leveraging our 'date' and 'close' columns.
for index , company in enumerate(tech_list , 1):
plt.subplot(2 , 2 , index)
filter1 = all_data['Name']==company
df = all_data[filter1]
plt.plot(df['date'] , df['close'])
plt.title(company)
Viz 1. Stock closing price change over 5 years for each company.
- These visualizations enable us to easily identify the highest peaks in each stock’s record, providing valuable insights.
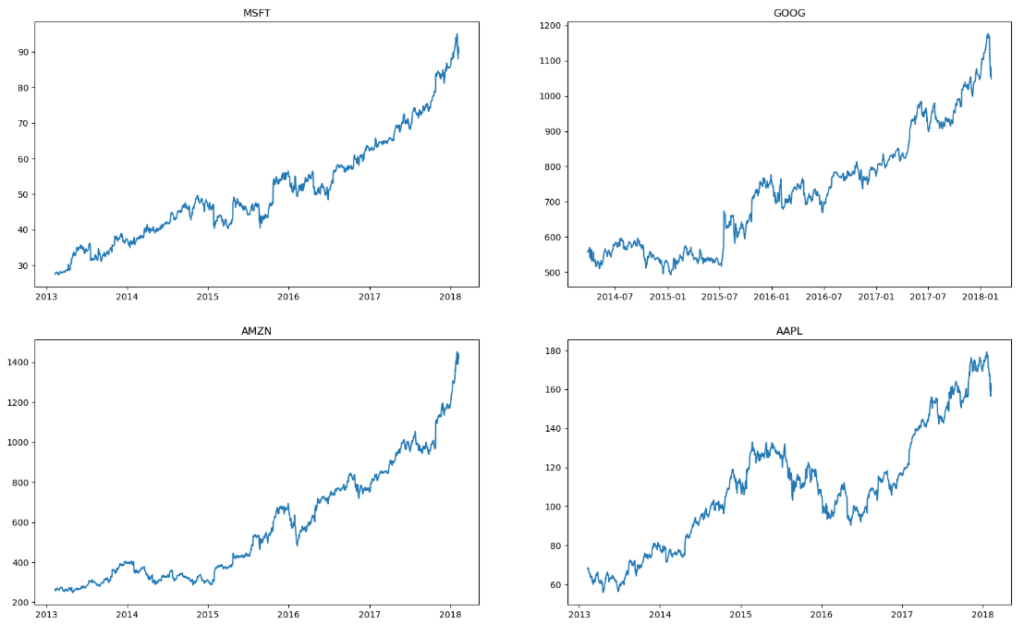
Now, an interesting question related to the stock market is: What were the moving averages of the various stocks?
all_data.head(15) # Here we are just exploring our columns, here we can see that we have two columns named 'high' and 'low' and will help us with the question above.
date open high low close volume Name
0 2013-02-08 27.3500 27.71 27.310 27.550 33318306 MSFT
1 2013-02-11 27.6500 27.92 27.500 27.860 32247549 MSFT
2 2013-02-12 27.8800 28.00 27.750 27.880 35990829 MSFT
3 2013-02-13 27.9300 28.11 27.880 28.030 41715530 MSFT
4 2013-02-14 27.9200 28.06 27.870 28.040 32663174 MSFT
5 2013-02-15 28.0400 28.16 27.875 28.010 49650538 MSFT
6 2013-02-19 27.8801 28.09 27.800 28.045 38804616 MSFT
7 2013-02-20 28.1300 28.20 27.830 27.870 44109412 MSFT
8 2013-02-21 27.7400 27.74 27.230 27.490 49078338 MSFT
9 2013-02-22 27.6800 27.76 27.480 27.760 31425726 MSFT
10 2013-02-25 27.9700 28.05 27.370 27.370 48011248 MSFT
11 2013-02-26 27.3800 27.60 27.340 27.370 49917353 MSFT
12 2013-02-27 27.4200 28.00 27.330 27.810 36390889 MSFT
13 2013-02-28 27.8800 27.97 27.740 27.800 35836861 MSFT
14 2013-03-01 27.7200 27.98 27.520 27.950 34849287 MSFT
all_data['close'].rolling(window=10).mean().head(14) # When calculating the moving average, it is useful to ->
# -> compute it in segments. We can consider groups of 10, 20, or 30 results, defined by our 'window' parameter.
0 NaN
1 NaN
2 NaN
3 NaN
4 NaN
5 NaN
6 NaN
7 NaN
8 NaN
9 27.8535
10 27.8355
11 27.7865
12 27.7795
13 27.7565
Name: close, dtype: float64
new_data = all_data.copy()
# We are now preparing our data so we can use Pandas to create a cool viz instead of using Matplot, just to have some fun.
ma_day = [10 ,20 , 50]
for ma in ma_day:
new_data['close_'+str(ma)] = new_data['close'].rolling(ma).mean()
new_data.tail(7) #This is just a quick trick so we can avoid those NaN resuls we got above
date open high low close volume Name close_10 close_20 close_50
4745 2018-01-30 165.525 167.3700 164.7000 166.97 46048185 AAPL 174.263 174.3340 172.9460
4746 2018-01-31 166.870 168.4417 166.5000 167.43 32478930 AAPL 173.096 174.0925 172.8726
4747 2018-02-01 167.165 168.6200 166.7600 167.78 47230787 AAPL 171.948 173.8700 172.8252
4748 2018-02-02 166.000 166.8000 160.1000 160.50 86593825 AAPL 170.152 173.2435 172.6356
4749 2018-02-05 159.100 163.8800 156.0000 156.49 72738522 AAPL 168.101 172.3180 172.3026
4750 2018-02-06 154.830 163.7200 154.0000 163.03 68243838 AAPL 166.700 171.7520 172.0640
4751 2018-02-07 163.085 163.4000 159.0685 159.54 51608580 AAPL 165.232 171.0125 171.7554
new_data.set_index('date' , inplace=True)
new_data
open high low close volume Name close_10 close_20 close_50
date
2013-02-08 27.350 27.71 27.3100 27.55 33318306 MSFT NaN NaN NaN
2013-02-11 27.650 27.92 27.5000 27.86 32247549 MSFT NaN NaN NaN
2013-02-12 27.880 28.00 27.7500 27.88 35990829 MSFT NaN NaN NaN
2013-02-13 27.930 28.11 27.8800 28.03 41715530 MSFT NaN NaN NaN
2013-02-14 27.920 28.06 27.8700 28.04 32663174 MSFT NaN NaN NaN
... ... ... ... ... ... ... ... ... ...
2018-02-01 167.165 168.62 166.7600 167.78 47230787 AAPL 171.948 173.8700 172.8252
2018-02-02 166.000 166.80 160.1000 160.50 86593825 AAPL 170.152 173.2435 172.6356
2018-02-05 159.100 163.88 156.0000 156.49 72738522 AAPL 168.101 172.3180 172.3026
2018-02-06 154.830 163.72 154.0000 163.03 68243838 AAPL 166.700 171.7520 172.0640
2018-02-07 163.085 163.40 159.0685 159.54 51608580 AAPL 165.232 171.0125 171.7554
4752 rows × 9 columns
new_data.columns
Index(['open', 'high', 'low', 'close', 'volume', 'Name', 'close_10',
'close_20', 'close_50'],
dtype='object')
plt.figure(figsize=(24,18)) # This for cycle works similarly as the last one we used to create the last viz, we are just using Pandas instead of matplot
for index , company in enumerate(tech_list , 1):
plt.subplot(2 , 2 , index)
filter1 = new_data['Name']==company
df = new_data[filter1]
df[['close_10','close_20','close_50']].plot(ax=plt.gca())
plt.title(company)
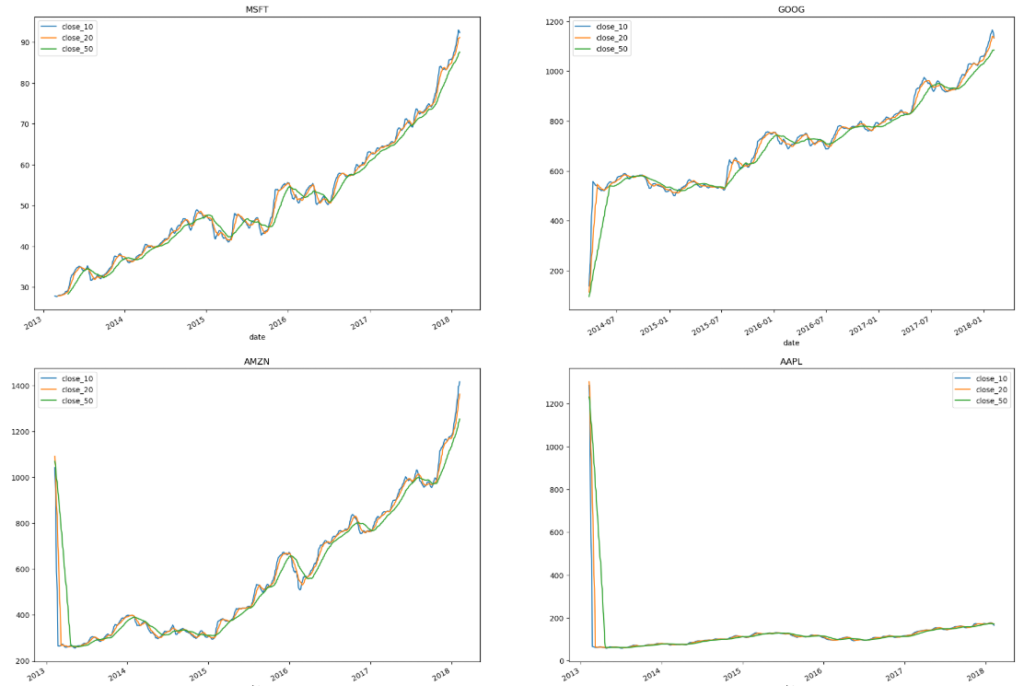
Viz 2. Stock moving average price change over 5 years for each company.
- These visualizations allow use to quickly compare different moving averages windows: 10, 20 and 50 days.
Let's analyze Apple closing prices change over time.
apple = pd.read_csv( r'C:\\Users\\Sergio C\\Desktop\\Analisis\\Stock analysis\\2-Time Series Data Analysis\\individual_stocks_5yr\\AAPL_data.csv')
apple.head(4)
date open high low close volume Name
0 2013-02-08 67.7142 68.4014 66.8928 67.8542 158168416 AAPL
1 2013-02-11 68.0714 69.2771 67.6071 68.5614 129029425 AAPL
2 2013-02-12 68.5014 68.9114 66.8205 66.8428 151829363 AAPL
3 2013-02-13 66.7442 67.6628 66.1742 66.7156 118721995 AAPL
apple['close']
0 67.8542
1 68.5614
2 66.8428
3 66.7156
4 66.6556
...
1254 167.7800
1255 160.5000
1256 156.4900
1257 163.0300
1258 159.5400
Name: close, Length: 1259, dtype: float64
apple['Daily return(in %)'] = apple['close'].pct_change()*100
apple.head(4)
date open high low close volume Name Daily return(in %)
0 2013-02-08 67.7142 68.4014 66.8928 67.8542 158168416 AAPL NaN
1 2013-02-11 68.0714 69.2771 67.6071 68.5614 129029425 AAPL 1.042235
2 2013-02-12 68.5014 68.9114 66.8205 66.8428 151829363 AAPL -2.506658
3 2013-02-13 66.7442 67.6628 66.1742 66.7156 118721995 AAPL -0.190297
import plotly.express as px
px.line(apple , x="date" , y="Daily return(in %)")
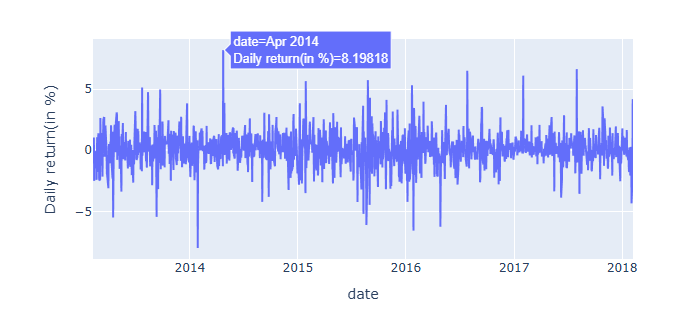
Viz 3. Apple stock change over time
- In this visualization, we observe several noticeable peaks over time. These peaks can be correlated with historical events, such as new product announcements.
Let's perform a resampling analysis.
What is resampling?
Resampling analysis is a statistical method that involves repeatedly sampling a dataset to estimate variability, test hypotheses, or aggregate data over specific intervals. Common techniques include bootstrapping, jackknifing, permutation testing, and time series resampling, often used in finance, forecasting, and model evaluation.
apple.dtypes # First we will make sure that data is under the format we need.
date object
open float64
high float64
low float64
close float64
volume int64
Name object
Daily return(in %) float64
dtype: object
apple['date'] = pd.to_datetime(apple['date'])
apple.dtypes
date datetime64[ns] #Here can see the changes.
open float64
high float64
low float64
close float64
volume int64
Name object
Daily return(in %) float64
dtype: object
apple.head(4)
date open high low close volume Name Daily return(in %)
0 2013-02-08 67.7142 68.4014 66.8928 67.8542 158168416 AAPL NaN
1 2013-02-11 68.0714 69.2771 67.6071 68.5614 129029425 AAPL 1.042235
2 2013-02-12 68.5014 68.9114 66.8205 66.8428 151829363 AAPL -2.506658
3 2013-02-13 66.7442 67.6628 66.1742 66.7156 118721995 AAPL -0.190297
apple.set_index('date' , inplace=True) # here we are just setting a different index to work easier with our data.
apple.head(4) #We can see that here we no longer have the first column and indexes have changed.
open high low close volume Name Daily return(in %)
date
2013-02-08 67.7142 68.4014 66.8928 67.8542 158168416 AAPL NaN
2013-02-11 68.0714 69.2771 67.6071 68.5614 129029425 AAPL 1.042235
2013-02-12 68.5014 68.9114 66.8205 66.8428 151829363 AAPL -2.506658
2013-02-13 66.7442 67.6628 66.1742 66.7156 118721995 AAPL -0.190297
apple['close'].resample('M').mean()
date
2013-02-28 65.306264
2013-03-31 63.120110
2013-04-30 59.966432
2013-05-31 63.778927
2013-06-30 60.791120
...
2017-10-31 157.817273
2017-11-30 172.406190
2017-12-31 171.891500
2018-01-31 174.005238
2018-02-28 161.468000
Freq: ME, Name: close, Length: 61, dtype: float64
apple['close'].resample('M').mean().plot()
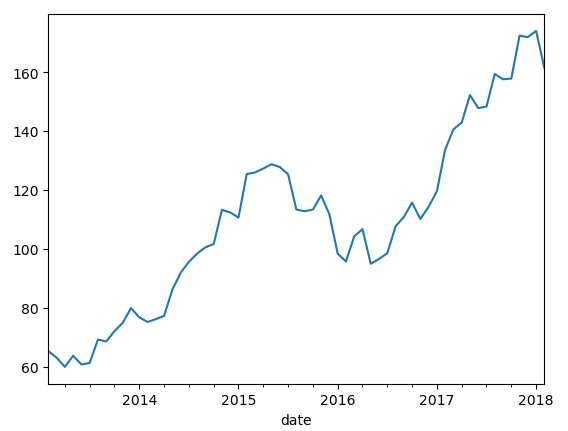
Viz 4. Apple change over time.
- In this visualization, we resampled our data on a monthly basis. The approach is flexible, allowing us to modify the code to generate similar results using different time windows, such as quarterly, weekly, or yearly intervals.
Are all these companies closing stock prices correlated?
Let’s perform a correlation analysis to identify relationships between stock price changes among the previously mentioned companies.
company_list
['C:\\\\Users\\\\Sergio C\\\\Desktop\\\\Analisis\\\\Stock analysis\\\\2-Time Series Data Analysis\\\\individual_stocks_5yr\\\\AAPL_data.csv',
'C:\\\\Users\\\\Sergio C\\\\Desktop\\\\Analisis\\\\Stock analysis\\\\2-Time Series Data Analysis\\\\individual_stocks_5yr\\\\AMZN_data.csv',
'C:\\\\Users\\\\Sergio C\\\\Desktop\\\\Analisis\\\\Stock analysis\\\\2-Time Series Data Analysis\\\\individual_stocks_5yr\\\\GOOG_data.csv',
'C:\\\\Users\\\\Sergio C\\\\Desktop\\\\Analisis\\\\Stock analysis\\\\2-Time Series Data Analysis\\\\individual_stocks_5yr\\\\MSFT_data.csv']
company_list[0]
'C:\\\\Users\\\\Sergio C\\\\Desktop\\\\Analisis\\\\Stock analysis\\\\2-Time Series Data Analysis\\\\individual_stocks_5yr\\\\AAPL_data.csv'
app = pd.read_csv(company_list[0])
amzn = pd.read_csv(company_list[1])
google = pd.read_csv(company_list[2])
msft = pd.read_csv(company_list[3])
closing_price = pd.DataFrame()
closing_price['apple_close'] = app['close']
closing_price['amzn_close'] = amzn['close']
closing_price['google_close'] = google['close']
closing_price['msft_close'] = msft['close']
closing_price
apple_close amzn_close google_close msft_close
0 67.8542 261.95 558.46 27.55
1 68.5614 257.21 559.99 27.86
2 66.8428 258.70 556.97 27.88
3 66.7156 269.47 567.16 28.03
4 66.6556 269.24 567.00 28.04
... ... ... ... ...
1254 167.7800 1390.00 NaN 94.26
1255 160.5000 1429.95 NaN 91.78
1256 156.4900 1390.00 NaN 88.00
1257 163.0300 1442.84 NaN 91.33
1258 159.5400 1416.78 NaN 89.61
1259 rows × 4 columns
sns.pairplot(closing_price)
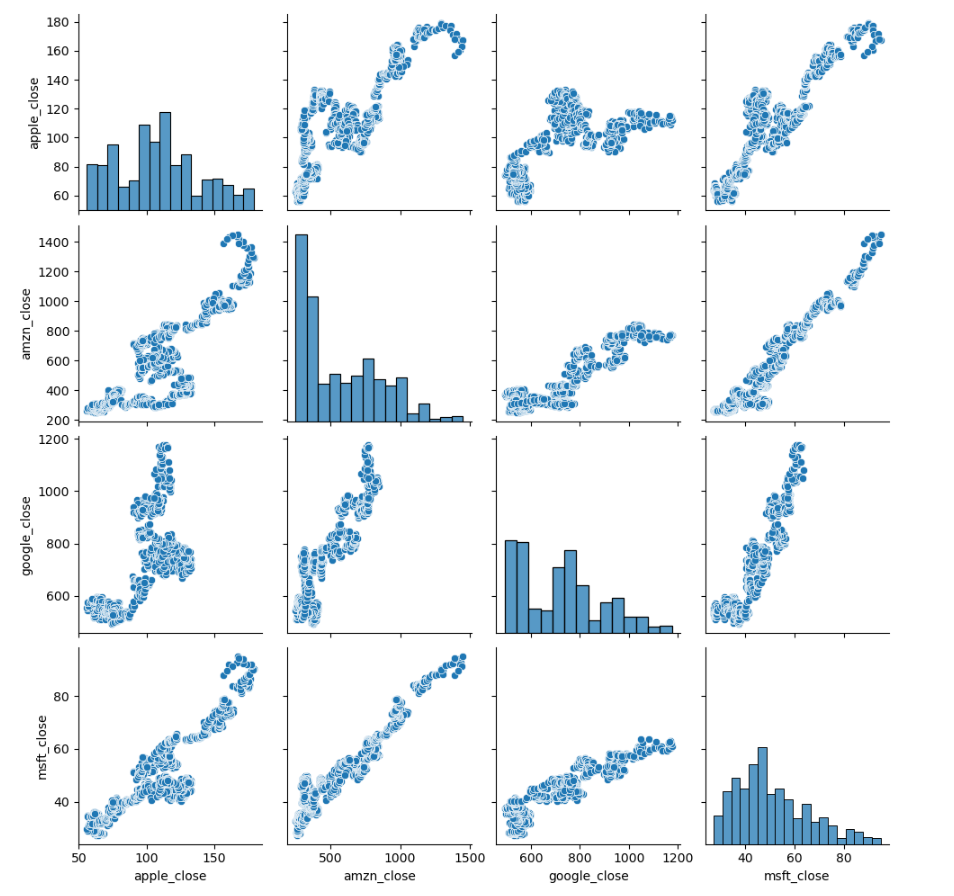
Viz 5. Correlation Pairplot.
- On this visualization we can easily notice that there’s a strong correlation between Microsoft/Amazon stock changes as they are the closer among x/y axis comparison.
But, how about a heatmap?
By adding some color to the mix, heatmaps make it easier to identify correlations among different concepts.
closing_price.corr()
apple_close amzn_close google_close msft_close
apple_close 1.000000 0.819078 0.640522 0.899689
amzn_close 0.819078 1.000000 0.888456 0.955977
google_close 0.640522 0.888456 1.000000 0.907011
msft_close 0.899689 0.955977 0.907011 1.000000
sns.heatmap(closing_price.corr() , annot=True)
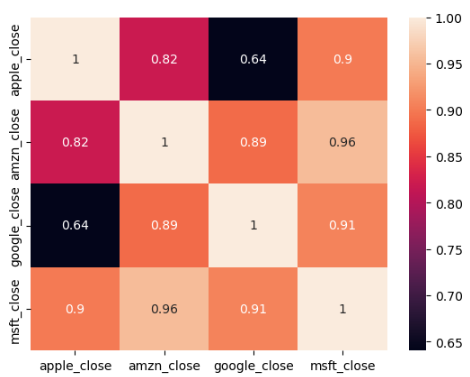
Viz 6. Correlation heatmap.
- On this visualization we can confirm that there’s a strong correlation between Microsoft/Amazon stock (0.96). Also, is easier to spot those that have no strong relation like the ones in black.
Let's end with a pairgrid.
closing_price
apple_close amzn_close google_close msft_close
0 67.8542 261.95 558.46 27.55
1 68.5614 257.21 559.99 27.86
2 66.8428 258.70 556.97 27.88
3 66.7156 269.47 567.16 28.03
4 66.6556 269.24 567.00 28.04
... ... ... ... ...
1254 167.7800 1390.00 NaN 94.26
1255 160.5000 1429.95 NaN 91.78
1256 156.4900 1390.00 NaN 88.00
1257 163.0300 1442.84 NaN 91.33
1258 159.5400 1416.78 NaN 89.61
1259 rows × 4 columns
closing_price['apple_close']
0 67.8542
1 68.5614
2 66.8428
3 66.7156
4 66.6556
...
1254 167.7800
1255 160.5000
1256 156.4900
1257 163.0300
1258 159.5400
Name: apple_close, Length: 1259, dtype: float64
closing_price['apple_close'].shift(1)
0 NaN
1 67.8542
2 68.5614
3 66.8428
4 66.7156
...
1254 167.4300
1255 167.7800
1256 160.5000
1257 156.4900
1258 163.0300
Name: apple_close, Length: 1259, dtype: float64
(closing_price['apple_close'] - closing_price['apple_close'].shift(1))/closing_price['apple_close'].shift(1)*100
0 NaN
1 1.042235
2 -2.506658
3 -0.190297
4 -0.089934
...
1254 0.209043
1255 -4.339015
1256 -2.498442
1257 4.179181
1258 -2.140710
Name: apple_close, Length: 1259, dtype: float64
for col in closing_price.columns:
closing_price[col + '_pc_change'] = (closing_price[col] - closing_price[col].shift(1))/closing_price[col].shift(1)*100
closing_price
apple_close amzn_close google_close msft_close apple_close_pc_change amzn_close_pc_change google_close_pc_change msft_close_pc_change
0 67.8542 261.95 558.46 27.55 NaN NaN NaN NaN
1 68.5614 257.21 559.99 27.86 1.042235 -1.809506 0.273968 1.125227
2 66.8428 258.70 556.97 27.88 -2.506658 0.579293 -0.539295 0.071788
3 66.7156 269.47 567.16 28.03 -0.190297 4.163123 1.829542 0.538020
4 66.6556 269.24 567.00 28.04 -0.089934 -0.085353 -0.028211 0.035676
... ... ... ... ... ... ... ... ...
1254 167.7800 1390.00 NaN 94.26 0.209043 -4.196734 NaN -0.789391
1255 160.5000 1429.95 NaN 91.78 -4.339015 2.874101 NaN -2.631021
1256 156.4900 1390.00 NaN 88.00 -2.498442 -2.793804 NaN -4.118544
1257 163.0300 1442.84 NaN 91.33 4.179181 3.801439 NaN 3.784091
1258 159.5400 1416.78 NaN 89.61 -2.140710 -1.806160 NaN -1.883280
1259 rows × 8 columns
closing_price.columns
Index(['apple_close', 'amzn_close', 'google_close', 'msft_close',
'apple_close_pc_change', 'amzn_close_pc_change',
'google_close_pc_change', 'msft_close_pc_change'],
dtype='object')
clsing_p = closing_price[['apple_close_pc_change', 'amzn_close_pc_change',
'google_close_pc_change', 'msft_close_pc_change']]
clsing_p
apple_close_pc_change amzn_close_pc_change google_close_pc_change msft_close_pc_change
0 NaN NaN NaN NaN
1 1.042235 -1.809506 0.273968 1.125227
2 -2.506658 0.579293 -0.539295 0.071788
3 -0.190297 4.163123 1.829542 0.538020
4 -0.089934 -0.085353 -0.028211 0.035676
... ... ... ... ...
1254 0.209043 -4.196734 NaN -0.789391
1255 -4.339015 2.874101 NaN -2.631021
1256 -2.498442 -2.793804 NaN -4.118544
1257 4.179181 3.801439 NaN 3.784091
1258 -2.140710 -1.806160 NaN -1.883280
1259 rows × 4 columns
g = sns.PairGrid(data= clsing_p)
g.map_diag(sns.histplot)
g.map_lower(sns.scatterplot)
g.map_upper(sns.kdeplot)
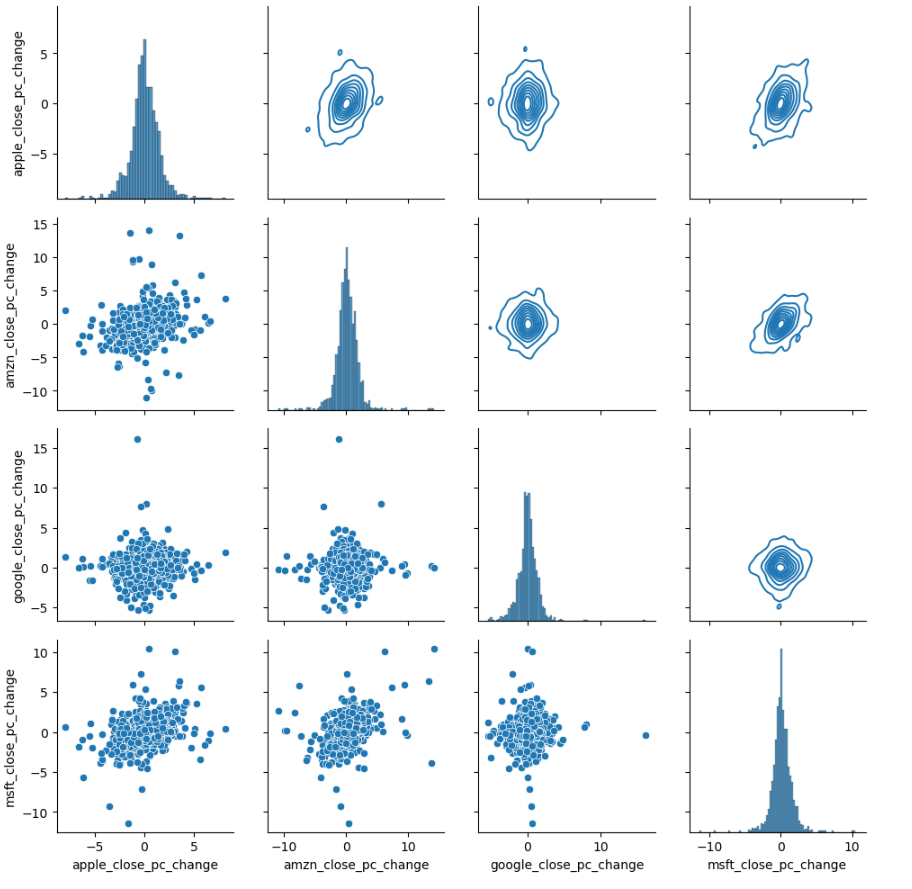
Viz 7. Pair Grid.
- This visualization allows us to compare different types of graphs, each providing unique insights into our data.
clsing_p.corr()
apple_close_pc_change amzn_close_pc_change google_close_pc_change msft_close_pc_change
apple_close_pc_change 1.000000 0.287659 0.036202 0.366598
amzn_close_pc_change 0.287659 1.000000 0.027698 0.402678
google_close_pc_change 0.036202 0.027698 1.000000 0.038939
msft_close_pc_change 0.366598 0.402678 0.038939 1.000000
Conclusions.
By considering the last prompt after closing_p.corr()
, we can observe different results. These results represent correlation scores between two companies’ stocks. For example, a score of 0.40 between MSFT and AMZN indicates a 40% likelihood that when one stock decreases, the other will also decrease. All the information and visuals obtained from this analysis provide highly valuable insights that can later be used to make informed decisions. Thank you for your time